
Big Data will Fuel Next Generation High End Machines

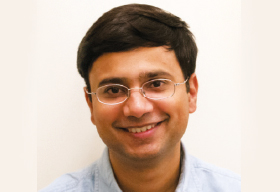
Ashish Thusoo, Co-Founder and CEO, Qubole Inc. , & Joydeep Sen Sarma, Co-Founder and CTO, Qubole Inc
What are the prospects that drive enterprises to harness Big Data? Why are most of the organizations getting stuck at the pilot stage of their big data initiatives?
Ashish: We are living in a time and age where there is a lot of data being produced. Machines are producing a lot of data that has created opportunities to harness it and create insights for products. The nature of the data has also changed; now it is a lot more unstructured and semi structured than it was before. So, because of this change big data has emerged. In enterprises today, these types of data can be harnessed to create different types of applications. However, it is a new and fast evolving industry; organizations do not have enough expertise to leverage the advantages of it. This has created an activation gap between what they want to do and their core capabilities. The activation gap essentially is defined by the use cases, they have users and they have demand for data and user data security, which is one side. On the other side IT budgets are failing constantly and more importantly their skill sets are very limited. Their skill sets are built on practices that were in use 20 years back. So that is the primary reason why despite the demand, a lot of these organizations get stuck even in the pilot stage.
The most obvious challenge associated with big data is simply storing and analyzing all that information. With most of the data being unstructured, managing it is a daunting task. What is the best way to address this challenge?
Joydeep: It is a very broad question. One of the biggest advantages of the way you tackle and structure data is that you are able to store it in much cheaper storage systems. The big data revolution actually started because we had the availability of Hadoop distributed file system which allowed enterprises to store large amounts of unstructured data in a significantly lower cost platform than traditional platforms of storing structured data. Same thing applies to cloud as well. With cloud I think it is better, so the first step to tackle this challenge is to store it in the right back end storage system.
When we were in Facebook and we built Hive, we built a system to put a catalogue on the unstructured data. The unstructured data became accessible to the entire organization. And that actually became a huge enabler for big data in the whole ecosystem. So that is the first layer what we call the access layer. Then we have the next layer that is about security. In Apache Hive and the big data ecosystem, there are bunch of different security solutions that provide granular security to data sets at table or file level and the column level.
The cloud vendor also has a very fantastic security story around the data. So, after that, people start asking questions around lineage. Like, where did this big data come from? How do I find out where it started, where it went? These are the kind of things that we are working on and the industry is working on. There are some partial solutions but general story here is that, as time goes on, you will see all of these things being built out.
When it comes to storage, businesses are resorting to converged and hyper-converged infrastructure and software defined storage. How does it help enterprises scale their hardware?
Ashish: I think there is a fundamental difference in the way architecture has evolved on premise as well as on the cloud. In on premise, you would get machines in the capex model and then suddenly people realize these machines we are using for storing the data have enough compute. So,let’s put the compute on top of these machines. When you come to the cloud, it is not a capex model, it is opex model. So you buy compute when you need it and remove when you don’t need it. Hence, the lifecycle of data or compute is separated out in the cloud. The cloud model has actually moved away from being converged to becoming diverged. So in the cloud, compute infrastructure is separated out from the storage infrastructure and that allows for a lot of automation and activity. None of the on-premise models could achieve that type of automation that we can achieve because of this divergence. So I would argue in the data world, divergence is much better than a hyper-converged architecture.
With recurring headlines on data breaches, the privacy and security issue has become a key concern. It can adversely affect the reputation of a company. What are the current glitches around data security?
Ashish: Data security is a complex topic. I think, in the last few months; there have been many data breaches. There are two things that have typically failed. Most fundamentally the failure is due to not adhering to certain policies, which leads to creating certain vulnerabilities in the system that is exploited by the folks. By and large, out of 100 cases, 99 would be pointed to process failure or a human failure, which led to somebody exploiting a technology failure. There is 1 out of those 100 cases which might be a technology failure.
Dealing with a shortage of talent in this field, enterprises are looking for analytics solutions with self-service or machine learning capabilities, designed to be used by professionals without a data science degree. How can such tools help organizations achieve their big data goals?
Ashish: One is lack of skills for running the platform; then there is also lack of skills for data science, folks who can use the platform. Obviously you have to produce more data scientists. The thing that is already happening is that there are verticalized applications which are encoding data science practices and creating a simpler interface for end user.
Joydeep: I totally agree. Let's take the example of Google which is great innovator in the data science and deep learning space but if you look at what they are trying to do, they have exposed much higher level of services. They realize that very few people are able to use sophisticated concepts and tools like deep learning and apply them. So for an average user, engineer or technically trained professional to be able to understand it is pretty hard. What is much easier is to give them higher level tools they can use to solve some end problem.
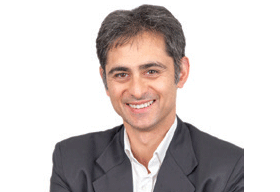
Ashish Thusoo
The variety associated with big data leads to challenges in data integration. What is the best way to address this challenge?
Joydeep: This was actually part of the genesis of the big data industry I would say. What people realized was that if you had one place where you could bring all of your data together. The industry started calling it a data lake. The infrastructure is already elastic and can scale almost infinitely, particularly in the cloud. The vendors are making it easier to process this data through better tools. So once you bring it all in, it is so easy to integrate data. The only major challenge that has emerged is security.
Is big data fruitful for big companies alone? How can SMEs leverage it for their benefit?
Ashish: I think big data is for any body who has got that big data problem. There are plenty of small and mid size companies who have big data problem. And the reason for that is that data collection now has become much easier.
Joydeep: People do not even realize that they are using big data. Everyone is benefiting from this big data platform, because all these SaaS platforms have a big data backing.
Ashish: I think there is a fundamental difference in the way architecture has evolved on premise as well as on the cloud. In on premise, you would get machines in the capex model and then suddenly people realize these machines we are using for storing the data have enough compute. So,let’s put the compute on top of these machines. When you come to the cloud, it is not a capex model, it is opex model. So you buy compute when you need it and remove when you don’t need it. Hence, the lifecycle of data or compute is separated out in the cloud. The cloud model has actually moved away from being converged to becoming diverged. So in the cloud, compute infrastructure is separated out from the storage infrastructure and that allows for a lot of automation and activity. None of the on-premise models could achieve that type of automation that we can achieve because of this divergence. So I would argue in the data world, divergence is much better than a hyper-converged architecture.
With recurring headlines on data breaches, the privacy and security issue has become a key concern. It can adversely affect the reputation of a company. What are the current glitches around data security?
Ashish: Data security is a complex topic. I think, in the last few months; there have been many data breaches. There are two things that have typically failed. Most fundamentally the failure is due to not adhering to certain policies, which leads to creating certain vulnerabilities in the system that is exploited by the folks. By and large, out of 100 cases, 99 would be pointed to process failure or a human failure, which led to somebody exploiting a technology failure. There is 1 out of those 100 cases which might be a technology failure.
Dealing with a shortage of talent in this field, enterprises are looking for analytics solutions with self-service or machine learning capabilities, designed to be used by professionals without a data science degree. How can such tools help organizations achieve their big data goals?
Ashish: One is lack of skills for running the platform; then there is also lack of skills for data science, folks who can use the platform. Obviously you have to produce more data scientists. The thing that is already happening is that there are verticalized applications which are encoding data science practices and creating a simpler interface for end user.
Joydeep: I totally agree. Let's take the example of Google which is great innovator in the data science and deep learning space but if you look at what they are trying to do, they have exposed much higher level of services. They realize that very few people are able to use sophisticated concepts and tools like deep learning and apply them. So for an average user, engineer or technically trained professional to be able to understand it is pretty hard. What is much easier is to give them higher level tools they can use to solve some end problem.
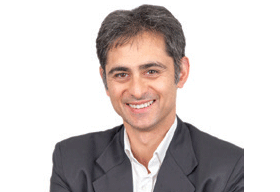
Ashish Thusoo
The variety associated with big data leads to challenges in data integration. What is the best way to address this challenge?
Joydeep: This was actually part of the genesis of the big data industry I would say. What people realized was that if you had one place where you could bring all of your data together. The industry started calling it a data lake. The infrastructure is already elastic and can scale almost infinitely, particularly in the cloud. The vendors are making it easier to process this data through better tools. So once you bring it all in, it is so easy to integrate data. The only major challenge that has emerged is security.
Is big data fruitful for big companies alone? How can SMEs leverage it for their benefit?
Ashish: I think big data is for any body who has got that big data problem. There are plenty of small and mid size companies who have big data problem. And the reason for that is that data collection now has become much easier.
Joydeep: People do not even realize that they are using big data. Everyone is benefiting from this big data platform, because all these SaaS platforms have a big data backing.